Congratulations to the REACH investigators listed below for their recent published work coming out of REACH!
- BACPAC REACH
- Sep 5, 2024
- 2 min read
Jessica Ornowski et al. published a paper titled, "Thresholding approaches for estimating paraspinal muscle fat infiltration using T1- and T2-weighted MRI: Comparative analysis using water-fat MRI". Following the comparison of four thresholding methods, we found that for all muscles combined, fat fraction (FF) measurements from T1- and T2-weighted images were strongly positively correlated with measurements from the water-fat images for all thresholding techniques (r = 0.70-0.86, p < 0.0001) and that variations in inter-muscle correlation strength were much greater than variations in inter-method correlation strength. We conclude that paraspinal muscle fat fraction can be quantified using T1- and T2-weighted MRI images with relatively low bias and absolute error in relation to water-fat MRI, particularly in the Multifidus and Erector Spinae. The choice of thresholding technique should depend on the muscle and clinical MRI sequence of interest.
Figure 1: Representative MRI images (upper) and muscle segmentations (lower) for an axial slice centered at the L2-L3 level from T1-, T2-, and IDEAL water–fat images. MF, ES, QL, and PS are highlighted as blue, green, yellow, and purple, respectively.
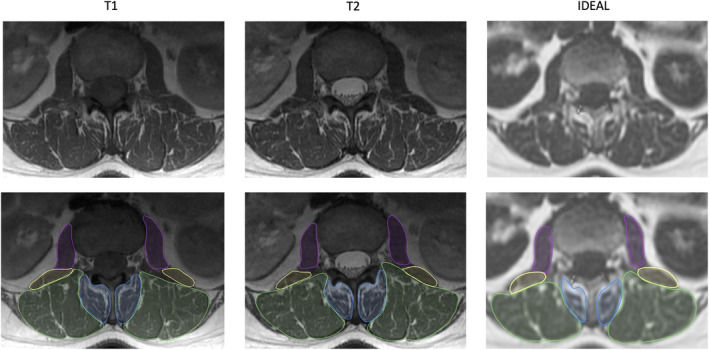
Read the full article in JOR Spine here.
Dr. Jeffrey Lotz et al. published a paper titled, "An Exploration of Knowledge Organizing Technologies to Advance Transdisciplinary Back Pain Research". Chronic pain conditions exhibit a hallmark of complex systems, the intertwining of manifold primary and secondary processes defying a unified theory and generalizable therapeutic approaches. That complexity is reflected in crosstalk, both in the condition, itself, and in the language of the research studying it. The BACPAC Theoretical Modeling Working Group (TMWG) developed a schema by which research and crosstalk can be organized. The work presented in this paper examines some technical requirements by which the knowledge represented in that schema (and elsewhere) might be formalized and challenged. There are 3 workflows laid out: prompt engineering for classification, clustering based on embedding space similarity, and the semi-automated extraction of structure from research texts. Each workflow incorporates the Open AI Large Language Model (LLM) called GPT. But they demonstrate use cases for GPT that go beyond the public conception and interface presented by ChatGPT. The extraction of structure from research language also incorporates Knowledge Graphs (KGs), which represents natural language as a network of subject-verb-object triples that can be reasoned over algorithmically. These concrete demonstrations present a sample of how these often opaque tools can be used.
Figure 6: The similarity graph visualizations of Schmid-64 references show green "h" nodes as hypothesis statements and blue "d" nodes as domain statements, with a ForceAtlas-2 layout used for the initial graph, and subsequent visualizations filtering or modifying nodes based on distance, co-authorship, or edge weights to highlight structural details and reference groupings.
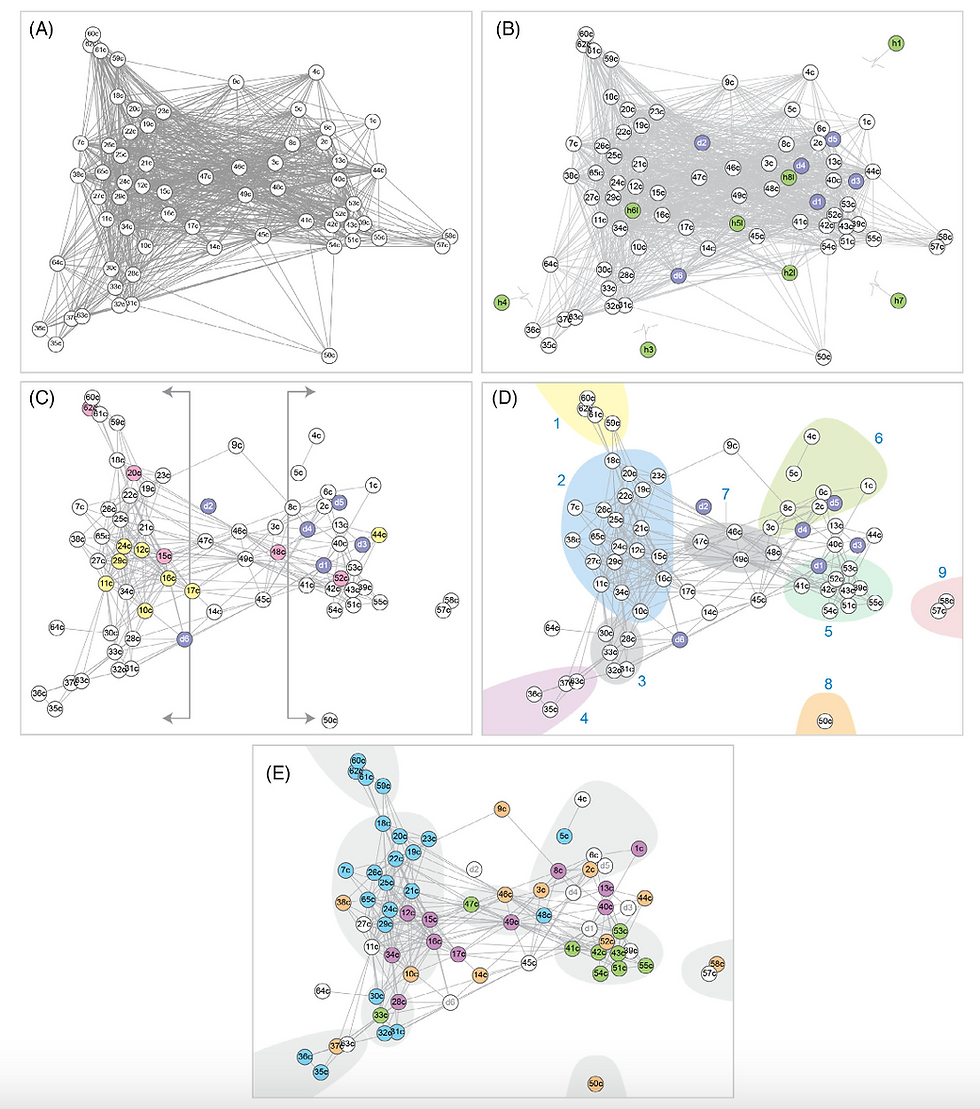
Read the full article on JOR Spine here.
Comments